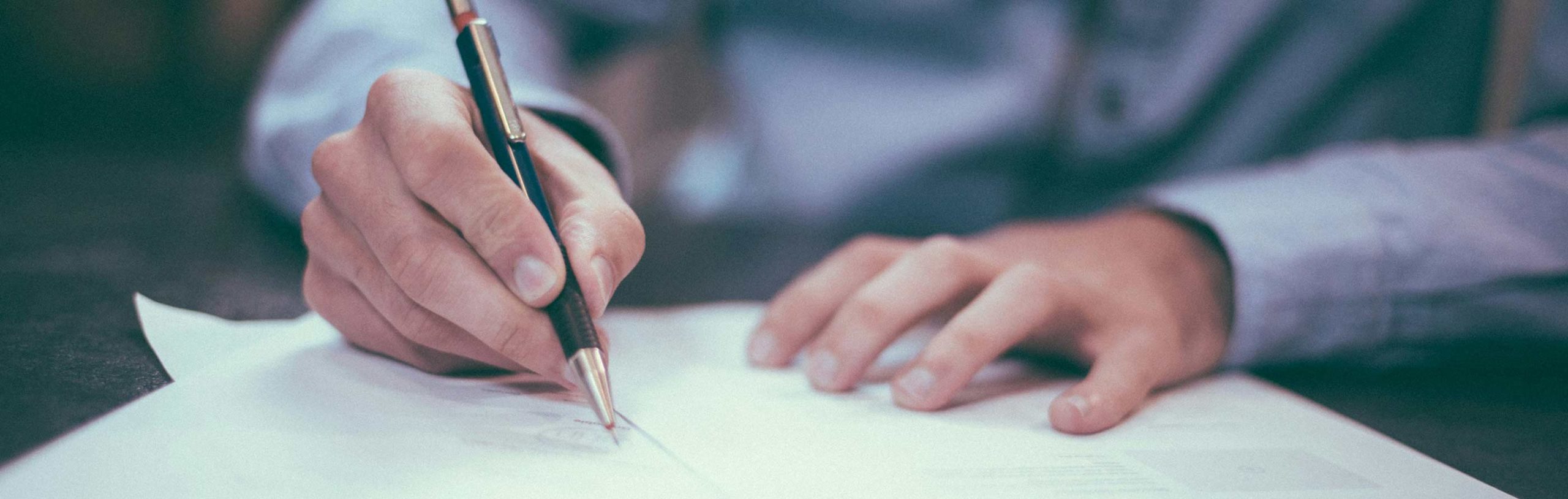
Best Practices in Healthcare MDM
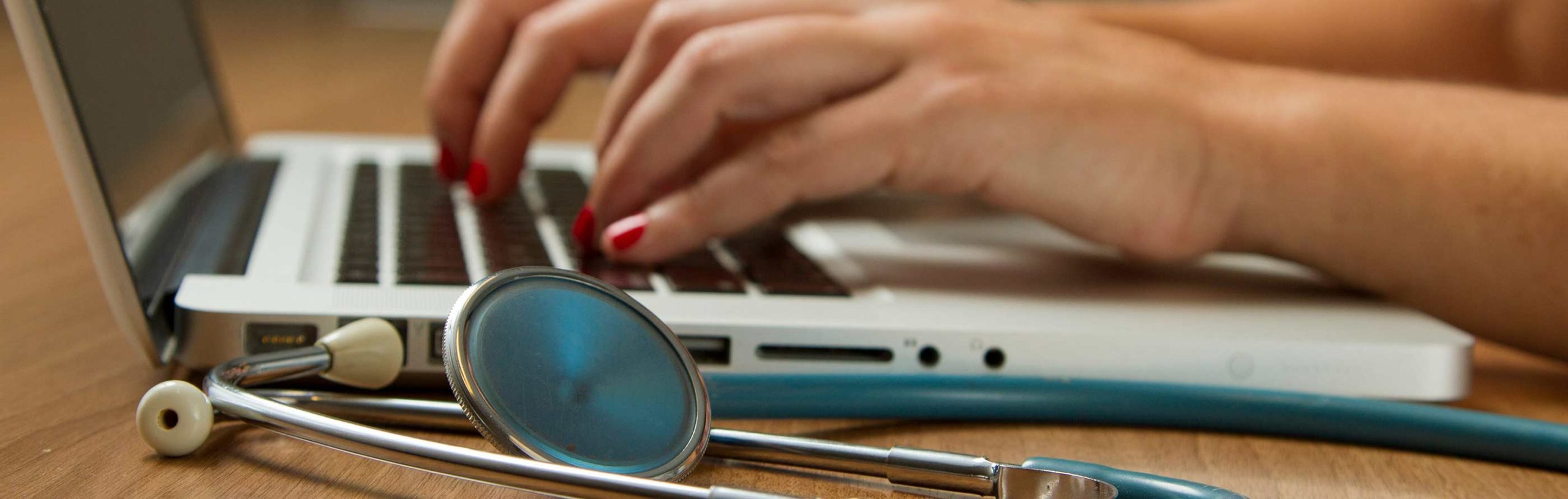
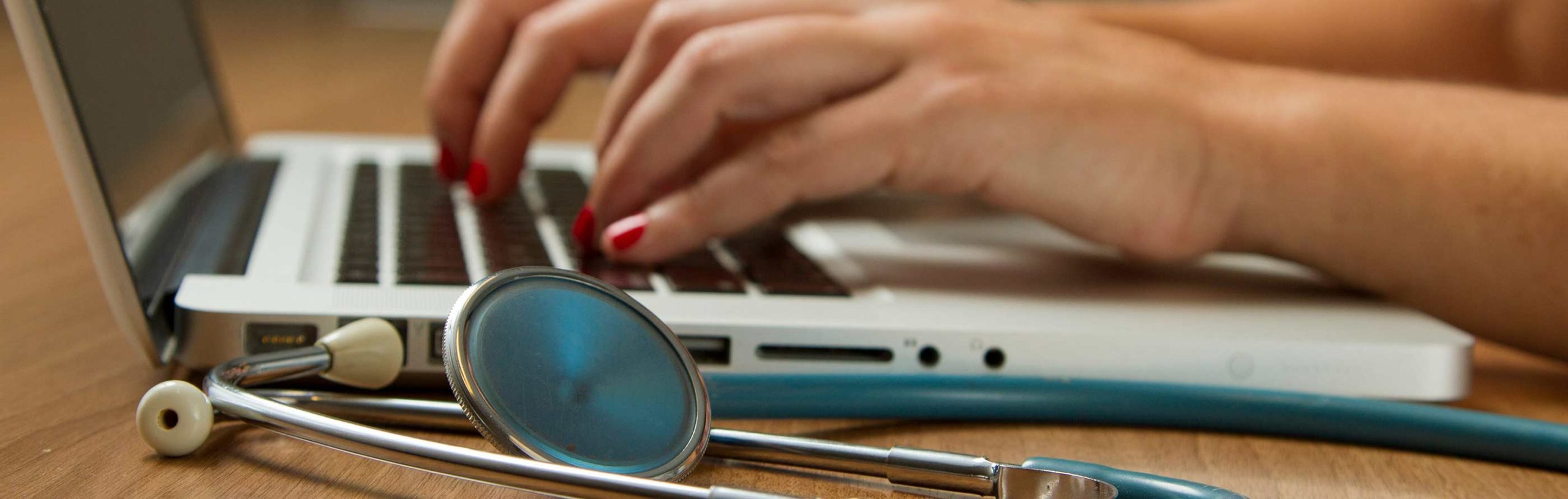
At Azulity, we empower our clients with a curated network of skilled professionals for their data needs. We offer data management services related to Data Governance, Master Data Management, Digital Transformation, Technology Implementation, and Program Management through our team of specialists across industries – including healthcare and financial services.
The healthcare domain, in particular, presents higher levels of complexities in the management of its data. This results in greater challenges for healthcare executives looking to implement and maintain high-quality Master Data Management or MDM, and leverage advanced predictive analytics for business insights.
In this issue, Brigitte Workman, our Director of Client Solutions, and resident expert in the field of MDM, specifically, Provider MDM, shares her real-world advice on implementing and governing MDM in healthcare organizations to ensure high data quality, and maximize ROI from analytics.
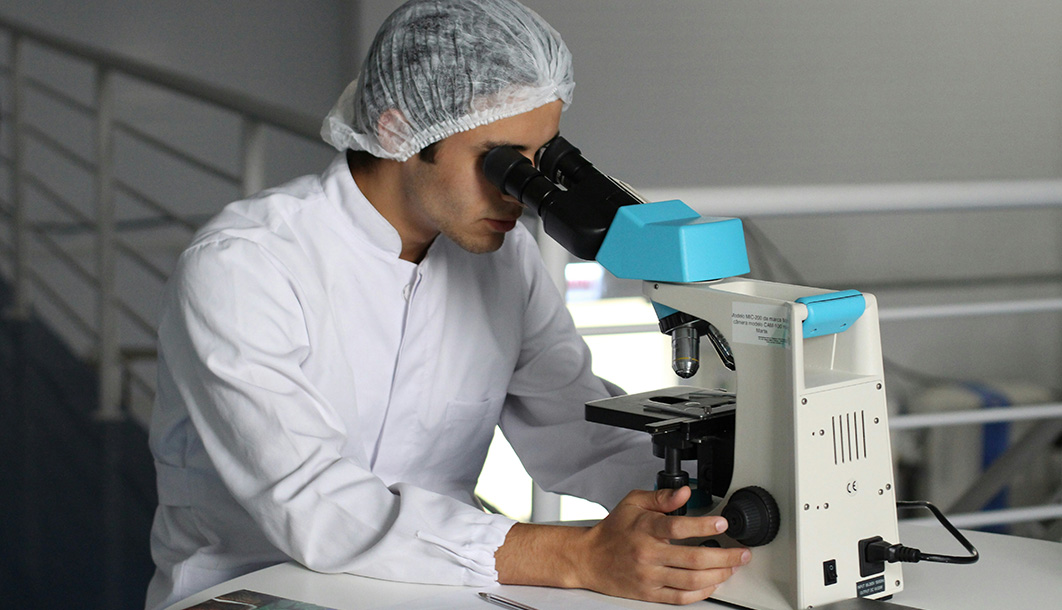
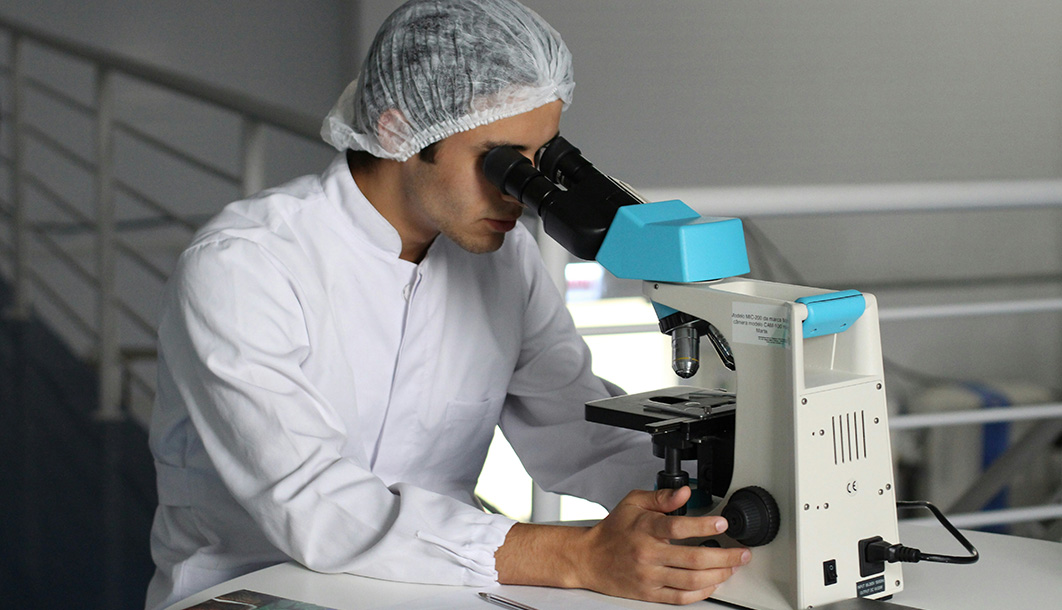
Best Practices In Healthcare MDM, by Brigitte Workman.
Health systems across the country are experiencing a paradigm shift in the way they operate. This is largely driven by an evolution in organizational culture – skewing towards enterprise-wide, data-driven decision making. Indeed, in a survey of over 200 providers and payer executives, 96% agreed that predictive analytics were important to the future of their business, with a majority dedicating 15% or more of their budgets to predictive analytics in 2019 [Society of Actuaries].
Predictive analytics in healthcare can deliver numerous advantages including risk stratification of patient health, and thereby the potential for early intervention, improvements in population health, and greater patient engagement. Additionally, on the operational side, it reduces administrative burden, and enables greater process efficiencies, including provider master data management.
The accuracy of predictive analytics is fueled by the quality of the underlying foundational data sets. In this regard, health systems struggle with sustaining quality levels for fragmented data sets, staying abreast of evolving data attributes, as well as updating and integrating legacy systems. In this era of big data, the sheer volume can seem burdensome to navigate.
How can health executives govern and successfully reap the benefits from their Master Data Management?
Implementing MDM Best Practices in Healthcare
The solution lies in prioritizing the management of the most critical assets to manage – particularly the backbone of improved analytics – the Master Data. This applies whether the data is related to patients, members, providers, supply chain, or other.
A common misconception is that a generic model of Master Data Management can work for healthcare. The reality is that healthcare data reflects the complexities and nuances of the entire healthcare system – brought about by greater regulations, laws, and the intricacies of navigating multiple evolving partnerships of providers, payers, patients, and other entities
If your organization is actively looking to develop Master Data, or fine-tune existing Master Data, consider the following recommendations for an asset needed by all healthcare organizations – the Master Provider Index. The nine points below are the absolute fundamentals required for the governance of Master Data Assets used across health systems:
1. Provider name (first, middle, last) – as displayed on state medical license(s)
2. Gender
3. Practicing degrees – (not education earned)
4. Practicing specialties – (not board certifications)
5. Status – by location
6. Categories of medical staff – (if applicable to populations in scope)
7. Source identifiers
8. Attributes used in match strategies (PECOS, NPI, and Personally Identifiable Information)
9. National Identifiers commonly used for certain provider populations (PECOS, NPPES, T-MSIS)
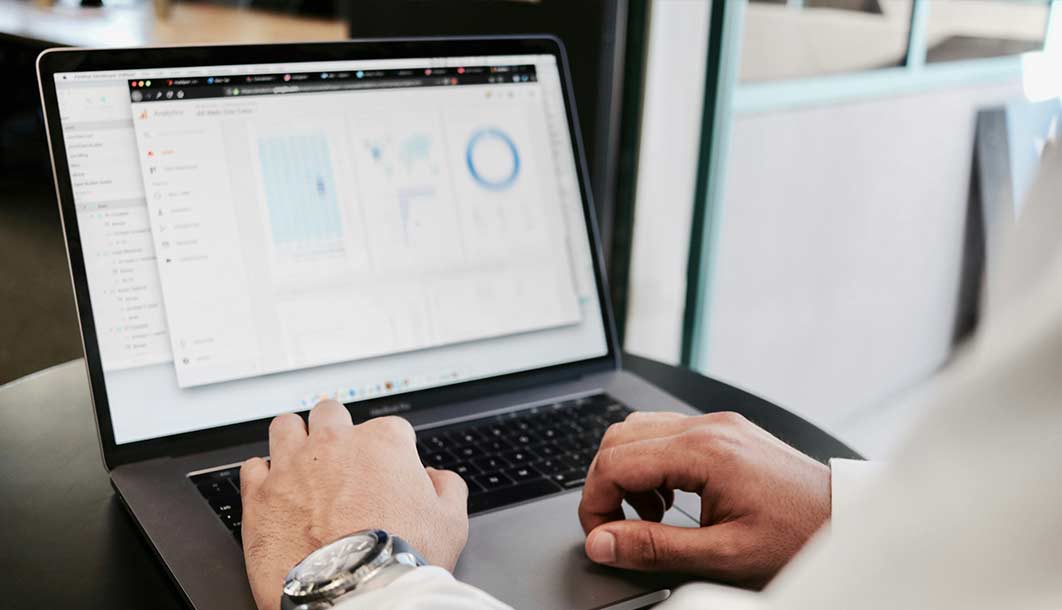
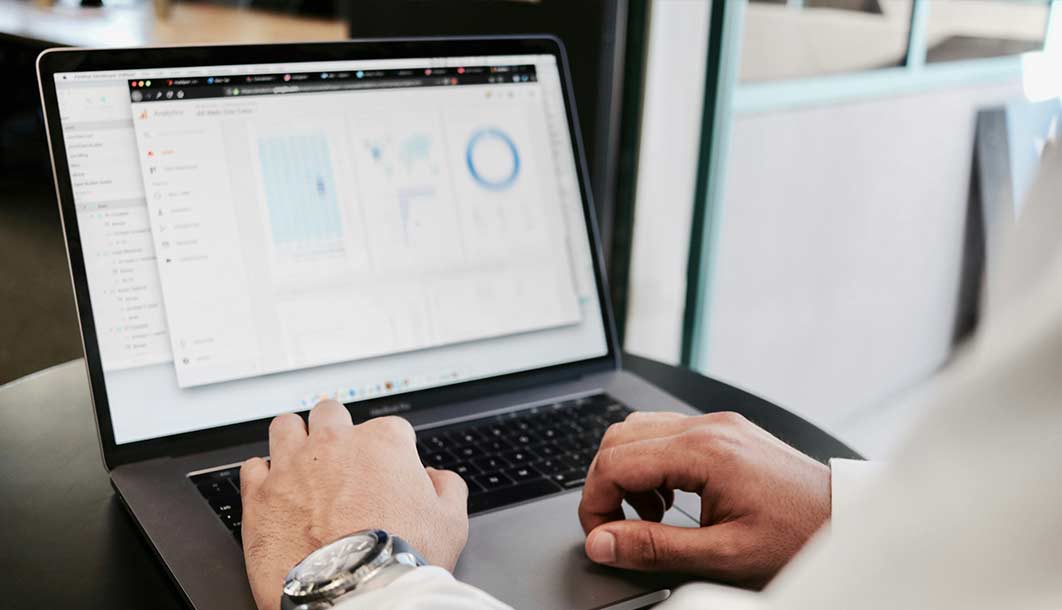
To set you and your teams up for success, and ensure the quality of your Master Data sets in both the short- and long-term, consider implementing the following steps:
Partner with Data Owners from key source systems
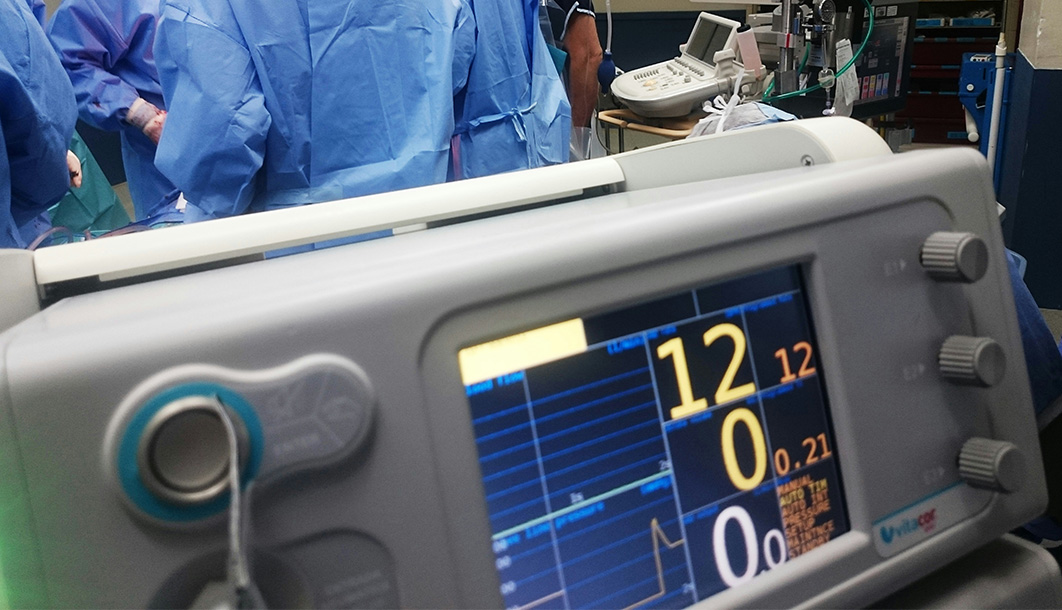
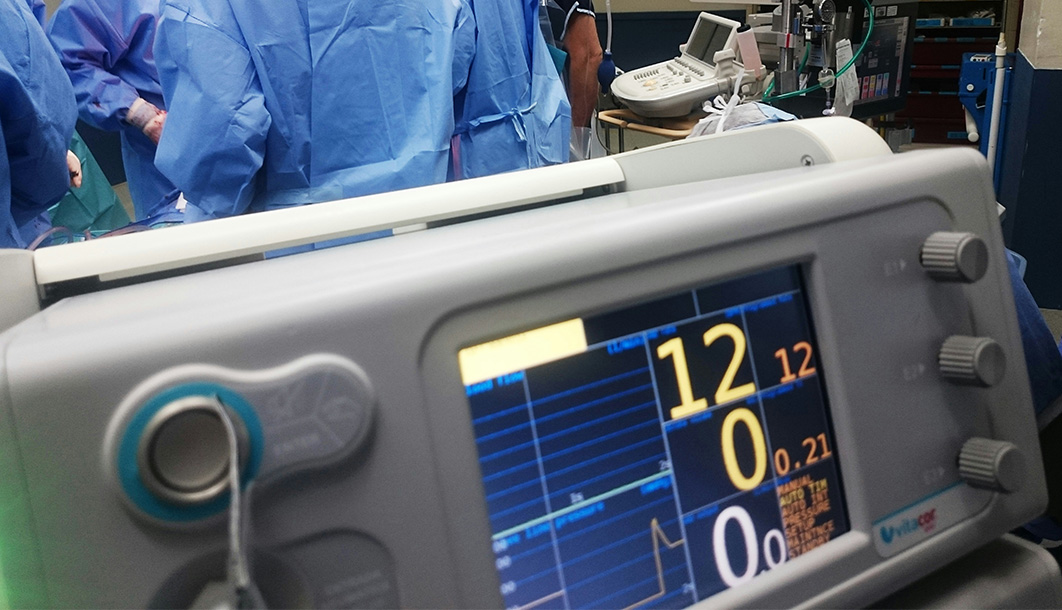
Data Owners refer to key organizational resources that maintain and utilize the fundamental assets listed above on a continual basis. These teams are also familiar with the source systems responsible for feeding these attributes to the Master Data. This includes Medical Staff Offices, Payer Enrolment/Contracting teams, Human Resources (for employed populations), Electronic Health Record teams, and more. These Data Owners can help identify strategic changes anticipated for source systems, enforce business rules for master data, and identify relevant Data Stewards.
Identify and Edify Data Stewards
Data Stewards, or guardians of an organization’s data, should be identified and appropriately trained under the direction of the Data or Source Owners. This will guarantee that the primary foundational data used is aligned to standards, thereby providing the best possible match rates, and the highest data quality. Data Stewards serve additional roles within the organization including the review of records when specific attributes require updates.
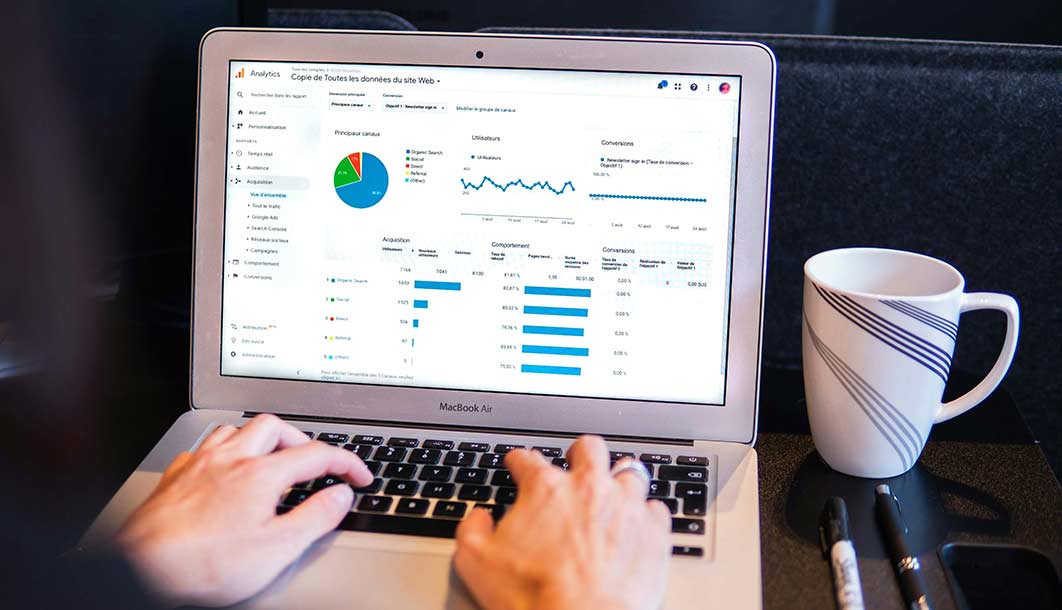
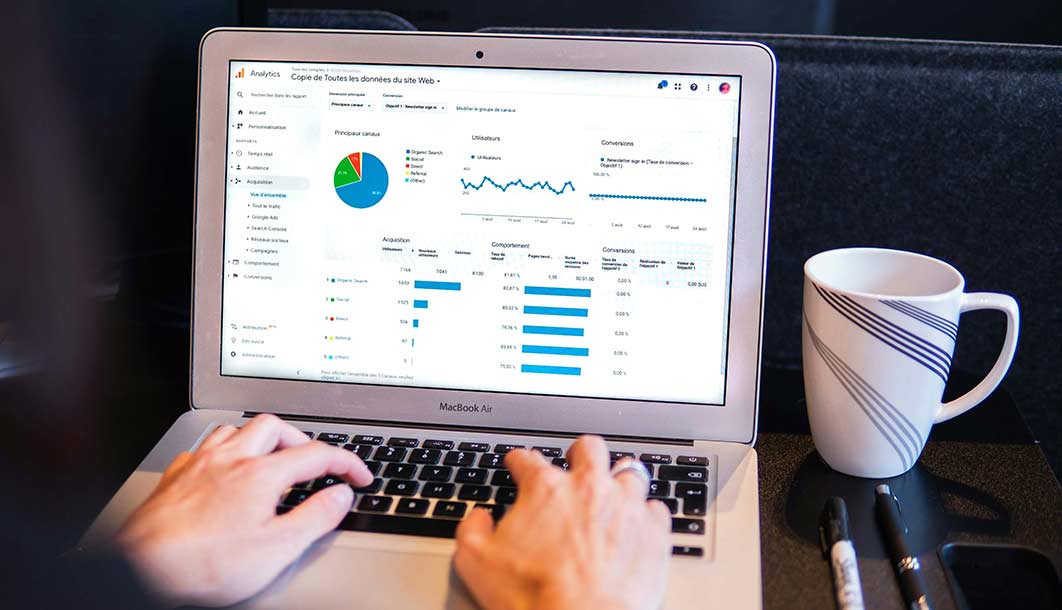
Develop Data Quality Dashboards
Develop a Data Quality Dashboard for the key attributes your organization will have to track on a continuing basis to ensure high data quality, and eventually, superior predictive analytics. Ideally, this step is conducted prior to even integrating the data sources. A dashboard provides information about how relevant source systems are populating the key attribute values with respect to time (fill rate), and if consistent – to maintain quality.
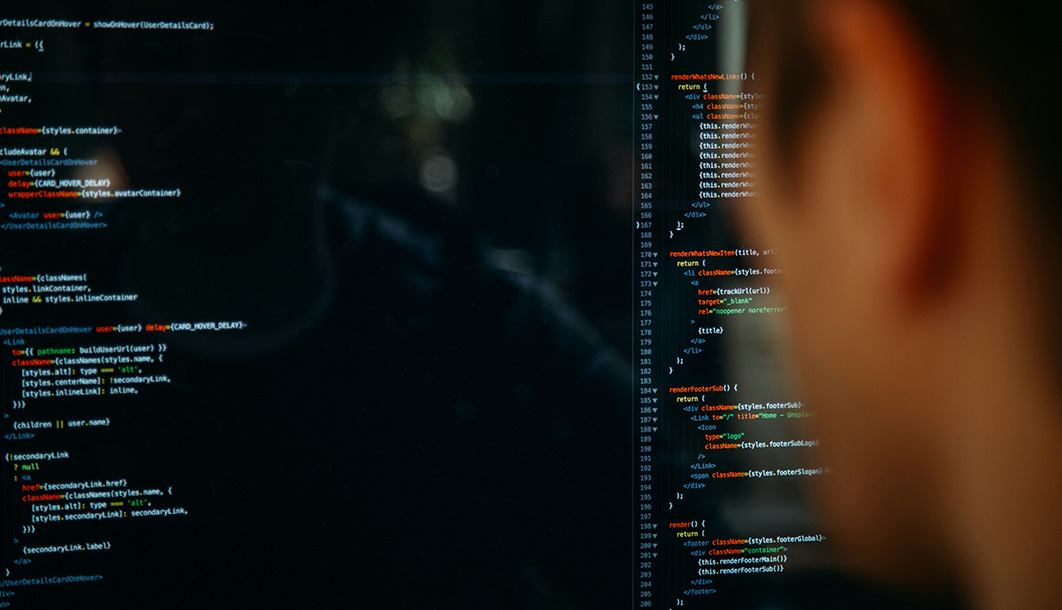
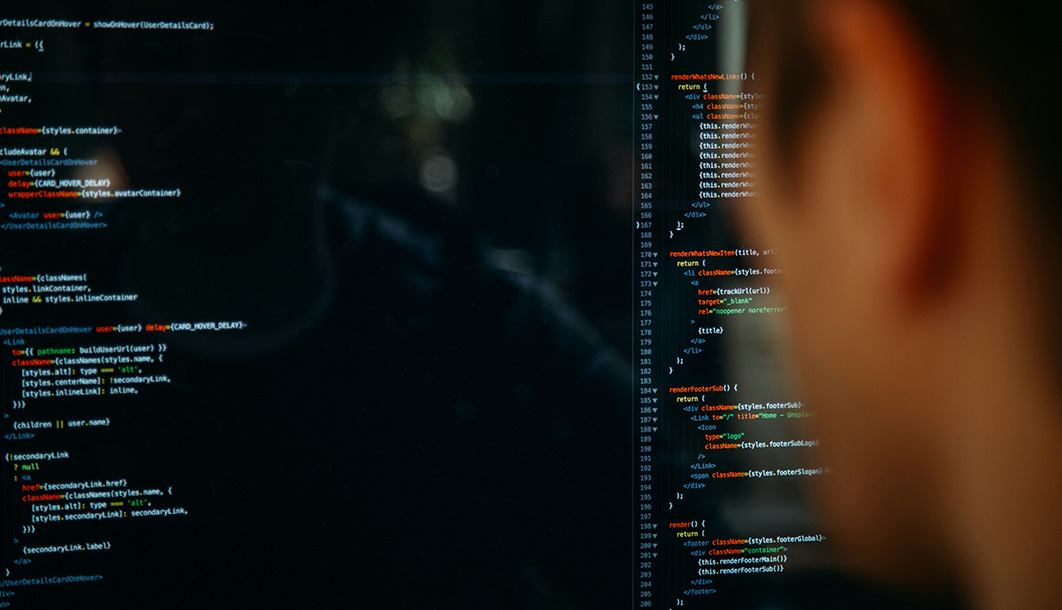
Communicate about Data Quality regularly
Communicate the results of the Data Quality Dashboard to the Data Stewards, Data Owners, and key stakeholders regularly. This will drive adoption across the organization. It also enables early detection of any potential issues, and timely course correction when required. Having developed the Data Quality Dashboard ahead of actual implementation of Master Data Initiatives also aids in obtaining buy-in from cross-functional teams, like business functions, at an early stage.
Waiting until after Master Data production is in progress burdens developers, and increases MDM operational overhead due to the management of match queues. Additionally, Data or Source Owners face added pressure to update records in a limited time period in preparation for the MDM go-live(s).
Conclusion
At first glance, the sheer volume of data involved may seem daunting to health systems looking to gain from quality data management initiatives and resulting predictive analytics. The key to governing the data is to prioritize and monitor critical attributes consistently, gather cross-functional support from Data Owners, select the right Data Stewards, and develop a Data Quality Dashboard even prior to initiating Master Data initiatives. These steps can go a long way towards realizing greater team efficiencies, reducing operational burden, and empowering a more effective path to managing Master Data.